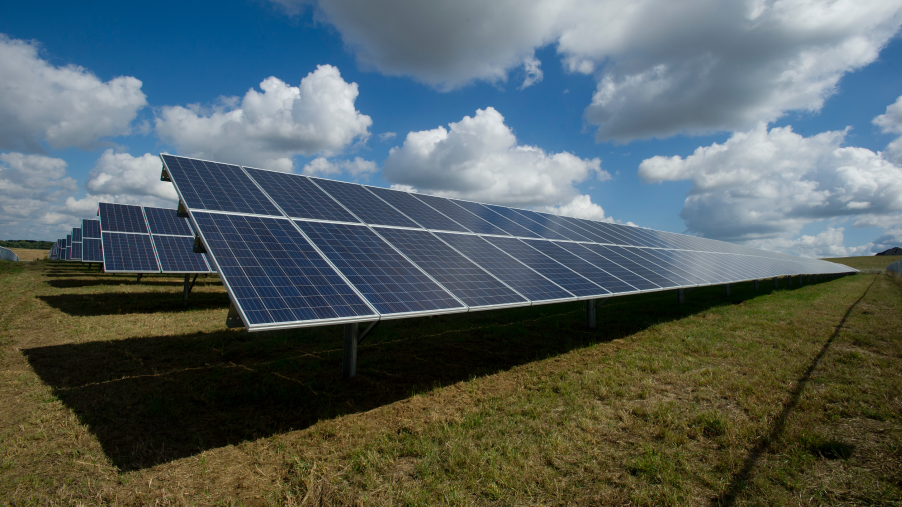
Solar is currently in the middle of exponential global growth, especially in the Middle East. The MENA region, already home to the world’s largest single-site PV plant, the 1.17GW Sweihan independent power project (IPP) in Abu Dhabi, is on an ambitious and rapid growth trajectory. Storage and Green Hydrogen are also expected to grow concurrently. This unprecedented growth will drive grids to evolve and adapt to a more decentralised, interconnected network of power generation and consumption.
Digital Twin, AI, Data and Digitalization will be required to manage these ever more complex systems for production, transportation and installation. Building Digital Twins at the installation stage and ensuring data is not siloed will allow for effective tracking of performance and degradation from Day 1 and continue to the Operations and Management (O&M) phase, so that power is produced smoothly for the entire life span, as the plant matures.
Traditionally, the solar industry has used “looking for the average” models using physics & math rules. These are static – and by its nature, a static model does not adapt and ignores actual operating conditions. Solar IPPs generally use SCADA data collection, spreadsheets and personnel to look at alerts and faults after they happen and perform analytics to compare with generation forecasts based on these models. Maintenance is generally based on fixed schedules, and issues are dealt with after they happen.
Digital Twin based models add inputs based on more substantial amounts of data and inputs from sensors. Using a model structure designed by Machine Learning (ML) experts leads to performance being improved by training with ever larger amounts of data arriving from an ever larger number of solar power plants. This process of Machine Learning is done over an extended time with careful selection of model structure and configuration parameters (hyperparameters) and thus immeasurably improves on the static models.
Digital Twins crucially improve solar assets for their owners and managers because Monitoring through Digital Twins also improves the asset quality of plants, as models are tuned to local environmental conditions and can optimise accordingly. Advantages of Digital Twins include;
Adaptability – Models can adapt to component tolerances, transportation and installation damage, local environmental concerns, geographic conditions, and changes in the nature of recurring faults.
Continuous Learning – Learn performance patterns driven by principles which are unknown to (or maybe even dismissed by) the model designer.
Degradation – Degradation is accounted for using hypothetical time travel; models can change their nature as the physical system ages or changes in environmental conditions.
With Digital Twins and AI, plants are now monitored predictively as opposed to reactively. Instead of simply monitoring for when a fault occurs and visualising the alert when it does, we move from preventative maintenance to proactive maintenance. The AI renders human error detection unnecessary for managing these larger portfolios. The AI, based on the Digital Twins, tracks signs & signals of impending issues in components. It then compares the impact of issues with revenue to issue work orders prioritised by revenue impact, ensuring that talented human capital spends less time on rectification and more on complex long term decisions. This automation and digitalisation of systems reduce the number of issues that occur over time as they are often solved before they happen, having a compounding effect on more careful inventory management for the whole portfolio. Lastly, the AI gets smarter and more accurate over time while running on the plant, reducing issues in the longer term.
Larger plants like those planned and built in the MENA region will require more focused technology resource planning for limited human resources handling ever larger portfolios. Here system-specific models will be more applicable than single, simple, idealised plant models rendering human error detection unnecessary for the asset management task. Specific characteristics of PV Plants already show that degradation leads to changes in their operational requirements and energy yield. This means adaptive models are needed for long term planning. With AI technology providing better tools, work teams are freed up to focus on minimising degradation over the lifetime of the plant. After all, these solar power plants are expected to remain operational & generate electricity for the next 30-40 years. Asset Owners and Independent Power Producers will also apply the qualitative learnings accrued by these adaptive Data based Digital Twins to the design phase of new projects, thereby creating a virtuous cycle of improvement.
These continuous learning and design guidelines utilising Digital Twins have been applied first to solar projects. The future development of Battery Storage and Green Hydrogen will require the same Digital Twins & Data solutions. We are already seeing Digital Twins maximising battery capacity, improving charge and discharge characteristics, improving availability during peak demand, and streamlining OEM claims. As more Green Hydrogen goes into production, we will see Digital Twins look at historical data and the interconnected relationships for rectifiers, electrolysis buffers, compressors and storage tanks. Technology vendors who can improve asset performance and apply lessons from Digital Twins to Battery Storage & Green Hydrogen will be ahead in MENA’s ever more competitive energy marketplace. Digital Twins & AI applied correctly reduces the concern of gird operators and consumers pertaining to the intermittent nature of solar power and maximises the capacity utilisation of these installations, making this collection of technologies an invaluable addition to the solar industry.
Comments are closed